Artificial intelligence creates enormous opportunities for businesses, but it also introduces risks traditional methods can’t fully cover. AI systems constantly change based on data, making them difficult to predict, manage, and control.
This article explains how AI risk management helps businesses recognize, understand, and proactively respond to risks unique to AI. You’ll discover how targeted risk management strengthens protection, accelerates innovation, simplifies regulatory adherence, and builds trust. You’ll also see how AI itself can streamline managing these challenges.
What is AI risk management and why is it important?
AI risk management helps your business identify and mitigate risks specific to artificial intelligence. Traditional methods rely on fixed rules and predictable results, but AI brings new challenges:
- AI models evolve rapidly and unpredictably.
- Poor or biased data leads to unreliable outcomes.
- AI decisions can be difficult to trace or understand clearly.
Specialized AI risk management addresses these complexities. It offers targeted guidelines built around the unique challenges AI presents, reducing uncertainty for your business.
Key benefits of proactive AI risk management
Proactive AI risk management gives your business:
- Enhanced protection: Identify vulnerabilities specific to AI before they become issues.
- Faster innovation: Deploy new AI products smoothly and without unexpected setbacks.
- Simpler regulatory adherence: Stay aligned with evolving rules like the EU’s General Data Protection Regulation (GDPR) or the California Consumer Privacy Act (CCPA) to reduce fines or legal complications.
- Increased transparency: Clearly understand AI decisions to make explanations easier for customers and regulators.
How AI risk management works in practice
Imagine launching an AI-powered chatbot for customer service. Soon after launch, customers complain about biased or inappropriate responses linked to hidden biases in your data. Your reputation takes a hit, and regulators investigate.
Proactive AI risk management prevents situations like these by evaluating data and models upfront, continuously monitoring AI interactions, and triggering instant alerts — helping protect your business reputation.
Top AI risks your business needs to manage
Businesses encounter distinct AI risks in several key areas. Effectively addressing these demands close cooperation across IT, compliance, legal, and business units. Understanding these risks early ensures smoother AI deployments.
Data risks
Data-related risks threaten the quality and safety of your AI systems. Here are some examples:
- Integrity issues: Outdated or incorrect data can lead to inaccurate AI outputs.
- Unauthorized access: Data breaches can expose confidential information.
- Privacy violations: Mishandling personal data can creat significant legal exposure.
For example, Microsoft researchers unintentionally exposed 38 terabytes of sensitive data in 2023 due to a misconfigured AI model. Similarly in 2023, facial recognition company Clearview AI was fined over 20 million Euros by several EU data protection authorities for scraping billions of facial images without user consent — a direct violation of GDPR regulations.
Beyond the astronomical penalties faced by businesses, these types of leaks can disrupt operations and, even worse, damage consumer trust.
Model risks
Model risks directly impact AI reliability in the following ways:
- Adversarial attacks: Hackers trick AI systems using deceptive inputs, which can result in incorrect predictions.
- Model drift: AI models lose accuracy over time as new data differs from initial training data.
- Prompt injection attacks: Malicious commands disguised as normal inputs can trick generative AI into harmful outputs.
- Algorithmic bias: Unrecognized biases in AI can lead to unfair outcomes.
Imagine your HR department implementing an AI-driven hiring tool that unintentionally discriminates against certain demographics due to biased training data. This can trigger compliance violations, lawsuits, and brand damage.
In the U.S., such bias may violate regulations enforced by the Equal Employment Opportunity Commission (EEOC), which prohibits workplace discrimination based on race, age, gender, and other protected characteristics.
Operational risks
Operational risks disrupt day-to-day AI reliability. Here’s what this looks like:
- Integration difficulties: Challenges connecting AI tools with current infrastructure have the potential to cause project delays.
- Unexpected downtime: System outages can interrupt critical services and business continuity.
- Scalability bottlenecks: AI systems can struggle with increasing workloads, creating slowdowns or failures.
- Long-term sustainability: Poorly planned AI solutions have the potential to become expensive or impossible to maintain over time.
Take financial teams, as an example. Poor AI forecasting can introduce compliance risks under regulations like the Sarbanes-Oxley Act (SOX), which requires accurate reporting and internal controls. Without strong oversight, even minor AI miscalculations could result in major financial discrepancies.
Regular system monitoring ensures your business catches operational problems early, maintains stable AI performance, and prevents costly disruptions.
Ethical and compliance risks
Ethical and compliance risks relate to fairness, transparency, and regulatory compliance requirements:
- Fairness issues: AI must treat all users equally and avoid biased results.
- Transparency gaps: Unclear AI decisions can complicate communication with users and regulators.
- Unclear accountability: Without defined responsibilities, AI governance has the potential to become fragmented.
A lack of clear governance can lead to significant business and legal consequences. For example, in 2022 the Irish Data Protection Commission (DPC) fined Meta Ireland 180 million Euros for breaches of the GDPR relating to Instagram and 210 million Euros relating to Facebook. The investigation found that Instagram and Facebook were using profiling, behavioral advertising, and more without disclosing to its users.
This example highlights the importance of responsible, transparent AI governance practices for your business.
Want to learn more about enterprise risk management? Learn how this strategic undertaking can help handle AI risks for your business.
The top AI risk management frameworks for enterprise organizations
Organizations often turn to proven frameworks designed specifically for AI governance. Each provides practical guidance to ensure ethical use, meet regulatory requirements, and mitigate emerging challenges effectively.
Here are some of the most widely adopted approaches:
The National Institute of Standards and Technology (NIST) AI Risk Management Framework (AI RMF)
The NIST AI RMF offers a versatile approach centered on 4 core functions:
- Govern: Set policies, roles, and accountability to oversee AI risks.
- Map: Identify AI systems and associated risks within your organization.
- Measure: Quantify AI risks based on potential impact and likelihood.
- Manage: Implement continuous monitoring and control strategies.
This framework supports clear decision-making, risk transparency, and responsible AI use at every stage.
International Organization for Standardization (ISO) /IEC 23894 Standard
ISO/IEC 23894 emphasizes transparency, accountability, and consistency across AI deployments. Intended for global businesses, it ensures uniform standards that facilitate regulatory adherence across multiple markets and regions.
European Union (EU) AI Act
The EU AI Act introduces a tiered approach to regulation, imposing stricter requirements on high-impact AI applications — especially those affecting human rights, safety, or privacy. Companies operating in Europe must demonstrate thorough governance, documentation, and transparency, making comprehensive AI controls essential.
Additional frameworks supporting responsible AI
Several other methodologies offer helpful guidance:
- Google’s Secure AI Framework (SAIF): Defines secure AI development practices, threat identification, and data protection methods.
- MITRE’s Sensible Regulatory Framework: Provides detailed threat modeling and specific regulatory guidance, especially useful for regulated industries.
- McKinsey’s AI Security Approach: Integrates AI governance within overall business objectives and company-wide risk tolerance.
These frameworks collectively reinforce global standards, ethical practices, and effective AI oversight — helping your business achieve consistent, reliable results.
4 steps to build an AI risk management framework
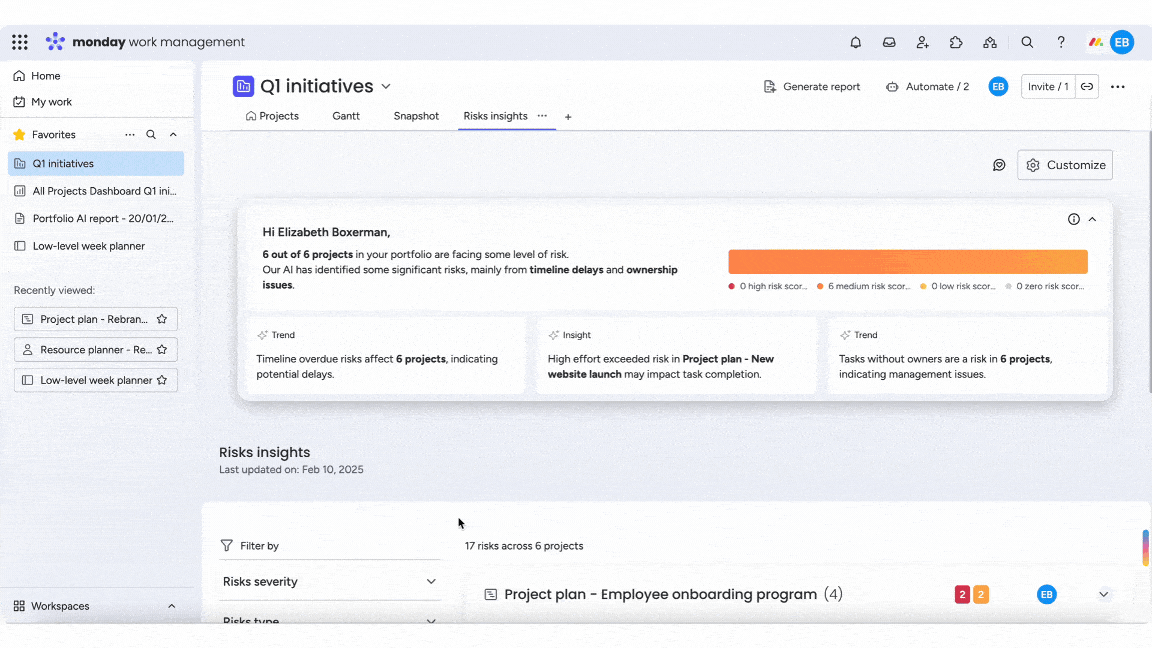
A well-defined AI framework provides your business with actionable steps to confidently handle challenges and align AI efforts with strategic goals. Follow these 4 steps for effective management:
1. Assess your AI risks
Start by understanding precisely where and how your organization uses AI:
- List every AI model, system, and data source clearly.
- Identify stakeholders responsible for oversight and operation.
- Prioritize potential risks using a simple impact-likelihood matrix.
Quick win: Use a straightforward spreadsheet or visual board that lists AI systems along with columns for owners, data sensitivity levels, known vulnerabilities, and potential impacts. This provides immediate visibility for your team.
2. Create an action-oriented governance plan
Convert your initial assessment into clear actions with defined accountability:
- Outline specific measures to address each identified issue.
- Establish roles, governance procedures, and direct accountability.
- Align your goals closely with business priorities and regulations like GDPR.
Quick win: Establish a concise checklist to:
- Assign accountability to specific stakeholders.
- Connect AI issues directly to relevant regulatory standards.
- Hold regular (monthly) reviews to maintain alignment.
3. Roll out AI solutions with controlled testing
Implement AI solutions systematically, reducing uncertainty through structured tests:
- Begin with small pilot implementations in controlled environments.
- Validate performance using scenario-based tests and compliance checks.
- Expand usage gradually, closely tracking performance and documenting outcomes.
Quick win: Conduct targeted pilots with clear success criteria, documenting results carefully before broader implementation. This minimizes unexpected outcomes.
Discover how to automate smarter, work faster, and make better decisions using monday AI Blocks, with a short lesson in monday academy. Learn how to use monday’s AI Blocks.
4. Monitor continuously with smart alerts
Maintain stable AI performance through continuous monitoring:
- Implement automated notifications that highlight issues immediately.
- Schedule periodic audits to verify ongoing model accuracy and performance.
- Adapt policies quickly in response to shifting regulations or business needs.
Quick win: Leverage AI-driven monitoring tools to automatically flag performance drops or unusual behavior, allowing your team to respond proactively.
Want to learn level up your enterprise AI risk management? Read the role of enterprise project management in strategic execution.
AI risk management templates for effective oversight
Templates simplify AI governance, ensuring consistent processes, saving time, reducing errors, and highlighting potential issues transparently.
Practical uses of AI risk management templates include:
- Clear documentation of AI inventory, risk assessments, and stakeholder responsibilities.
- Consistent evaluation methods to prioritize risks based on severity and business impact.
- Industry-specific adaptation — templates tailored for finance, healthcare, technology, and more, each addressing unique regulatory and operational requirements.
Example of an AI risk management template in action
AI system | Owner | Data sensitivity | Potential risks | Risk level | Relevant regulations | Mitigation steps | Review frequency |
---|---|---|---|---|---|---|---|
HR hiring tool | Steve Roberts (HR) | High (personal) | Bias in training data | High | GDPR, EEOC | Regular bias audits; Retrain model quarterly; Use diverse data | Monthly |
Customer chatbot | Cornelia Brown (CX) | Medium (customer interactions) | Offensive outputs, data leaks | Medium-high | GDPR | Daily log reviews; AI sentiment monitoring; Immediate alerts for flagged outputs | Weekly |
Forecasting engine | Sarah Roth (Finance) | High (financial records) | Inaccurate forecasts, data input errors, model drift | Medium | SOX, GDPR | Regular model validation; Cross-check with manual forecasts; Update data quarterly | Quarterly |
With monday work management, structured templates integrate directly into your workflow. Easily track AI risks, clearly assign tasks, monitor progress continuously, and simplify compliance management — helping your team proactively manage risk and move forward with confidence.
Avoid AI risks across your organization with monday work management
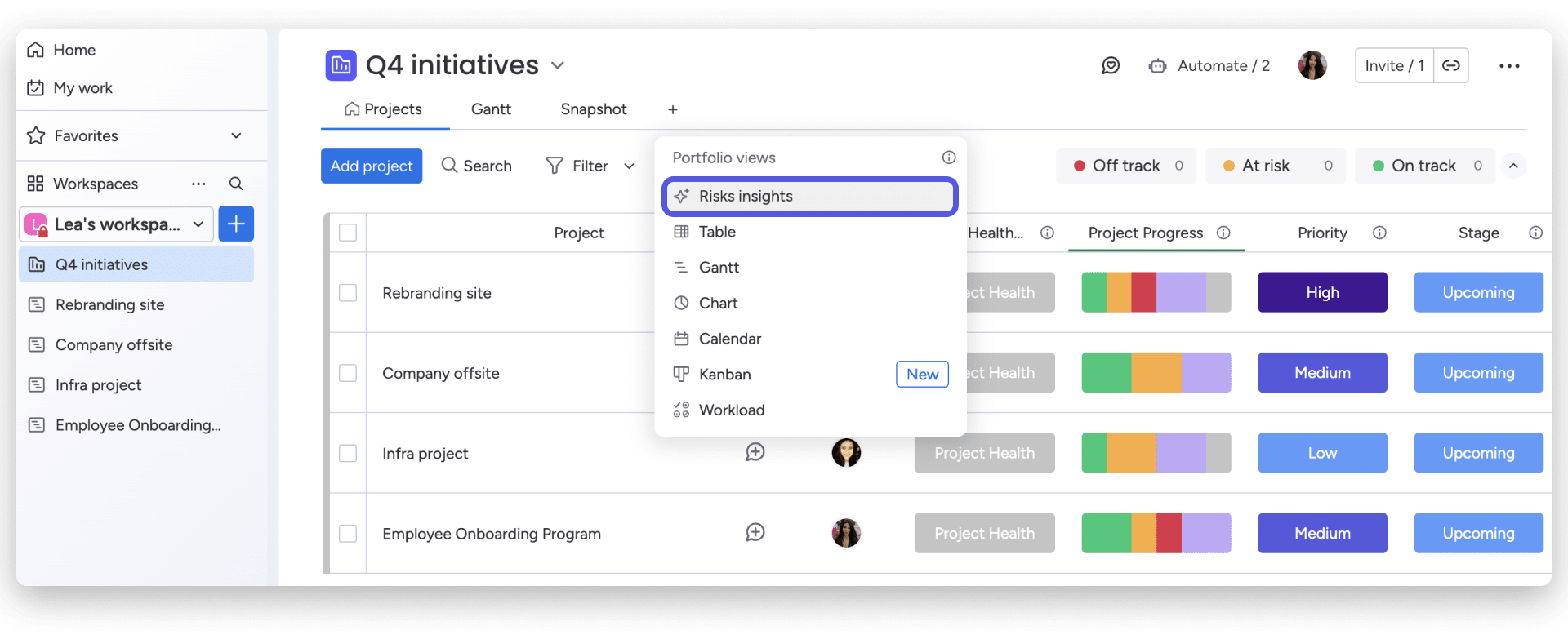
Managing AI risks manually can quickly drain your team’s time, create mistakes, and slow down progress. You need a streamlined approach that spots issues early and keeps your projects running smoothly.
Enterprise-level AI risk handling becomes simpler with intelligent automation from monday work management. Here’s how it streamlines your team’s workflow:
- Instant issue detection: Portfolio Risk Insights scans all your project boards, quickly flagging potential AI risks by severity. Spot critical issues at a glance, without manually combing through data.
- Continuous, actionable alerts: Get immediate updates as new risks emerge, so your team stays ahead of problems. Instantly know which projects need urgent attention.
- Quick notifications to project owners: With just a click, notify the right person about an emerging risk. The platform generates a clear summary and links relevant tasks, helping your team jump into action.
- Executive-ready AI reports: Easily generate daily reports that highlight trends, risks, and project health. Visual dashboards keep leadership informed, making strategic decisions straightforward.
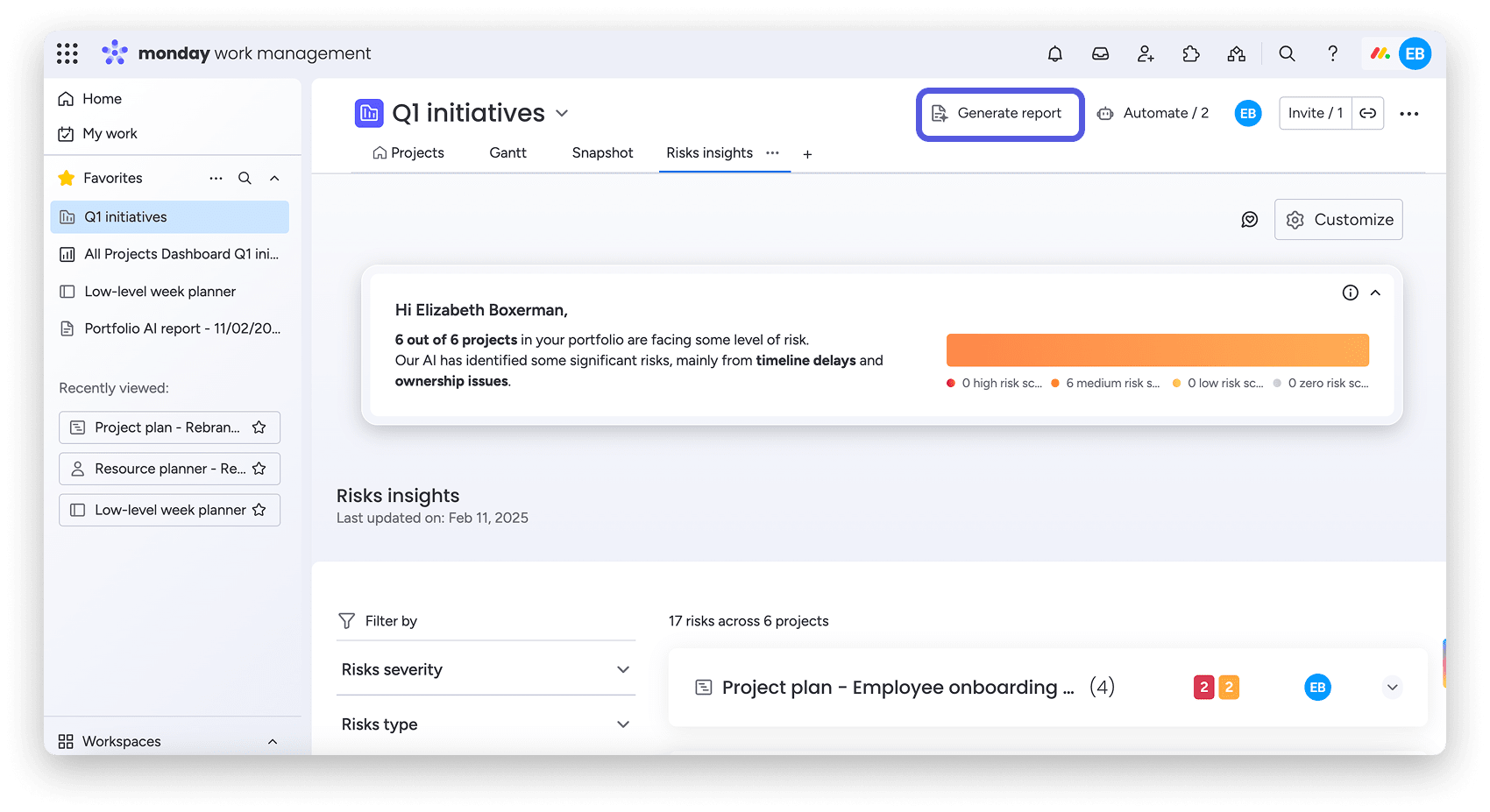
Enterprise teams rely on monday work management to:
- Eliminate manual busywork and reclaim time.
- Address risks early before they become larger disruptions.
- Improve accountability by defining risk management responsibilities.
With monday work management, AI risk management becomes intuitive, automatic, and efficient — so your team can confidently drive projects forward.
Want to learn more about how AI can help manage projects beginning to end? Read about the power of AI in project management.
Turn effective AI risk management into your competitive advantage
Effectively managing AI challenges protects your business, accelerates innovation, and ensures you remain aligned with regulatory requirements. Companies that leverage smart governance frameworks stay ahead in the competitive landscape.
Complex manual processes become easy-to-follow workflows through monday work management. With Portfolio Risk Insights, your team can consistently identify, categorize, and clearly communicate emerging issues — helping you maintain compliance and proactively manage threats.
Make intelligent, proactive AI governance your advantage with monday work management.
FAQs
How is AI used in risk management?
AI is used in risk management to identify and manage risks using predictive analytics, anomaly detection, automated risk assessments, and instant reporting. These tools enable businesses to spot potential problems early and respond quickly.
Will risk management be replaced by AI?
No, AI won’t fully replace risk management professionals. Human judgment remains essential for understanding context, ethical concerns, and critical decisions. AI complements human efforts by automating routine tasks and highlighting critical risks.
What companies are using AI for risk management?
Examples of companies using AI for risk management include JPMorgan Chase, IBM, and Google. They are using AI to predict financial risk, ensure compliance, and protect data. Additionally, industries such as finance, healthcare, insurance, and technology actively use AI in risk management.
Can AI predict risk?
Yes, AI effectively predicts many risks through data analysis and pattern recognition. Although it excels at forecasting trends and highlighting potential threats, AI can't perfectly predict all events due to uncertainty and unexpected variables.
What is the biggest risk of AI?
The biggest risk of AI is the potential for biased or incorrect outcomes resulting from flawed data or algorithms. These errors can cause significant reputational damage, compliance violations, and unintended harm to users.
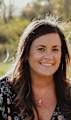